A few weeks ago I was hiking in Bryce Canyon with my family–including Nora, my 3 (and 1/2) year old great-niece. We had some delightful conversations–including the following (a bit of artistic liberty taken here):
Me (to Nora’s dad): Which way are we going back?
Nora: Melissa, you have to go left, right, right, and then left. I read the map.
Me (to Nora’s dad): Does she already know left and right?
Nora’s Dad: She has a lot in common with ChatGPT.
Nora’s Dad (to Nora): When did you learn to read maps?
Nora: When I was 1.
We laughed. And turned right.
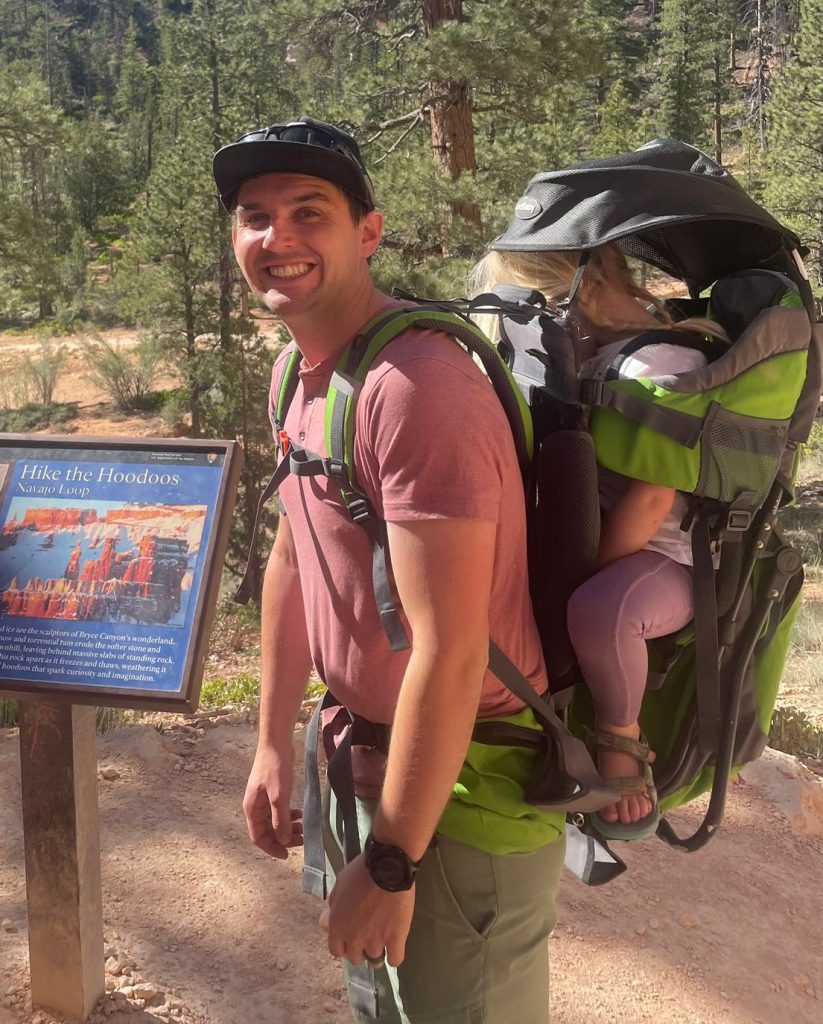
What respectable academic studying GenAI would not over-analyze this conversation? Here we go.
First, a Word About Metaphor
There is, appropriately, concern about anthropomorphizing AI, and the types of thinking errors this can cause. This post will, admittedly, use a lot of anthropomorphized language because it is hard to avoid.
Ultimately, our tendency to anthropomorphize is a metaphor–thinking of a non-human as a human. In a recent post, Punya Mishra, Nicole Oster, and I discussed how metaphors are what we use to think and communicate, to understand and experience the world. Leon Furze also recently wrote about this topic. Metaphors are not perfect, but they are the only way we have to make sense of things. My hope is that encouraging thinking through multiple metaphors can help us take what is useful from each and reject what is not.
So this post, like all language, has a lot of metaphors–none are perfect representations, all have flaws and shouldn’t be taken literally, but each can provide a little bit of insight to shape our thinking about something new and strange.
Metaphor 1: The very label “Artificial Intelligence“. Let’s skip (i.e., playfully hop over) this one.
Metaphor 2: Hallucinate. We have begun to commonly use this term when AI says something false. But, as Punya Mishra argues, it is always hallucinating–that is all it does. So…is it really hallucinating, or is it doing something else? Hallucinate is a metaphor we are using to understand AI, but, like the metaphors I will discuss next, it is not perfect. But, alas, it is all we have. Here we go with another.
Metaphor 3: ChatGPT and 3 Year Olds
Back to Nora. Nora is 3 1/2. She is brilliant (I’m not biased). But she hasn’t quite mastered cartography (she’s going to be disappointed when her baby brother fails to read his first map at age 1).
So–was Nora “hallucinating”? Was she lying? Pretending? Or was she innocently putting together words she hears in a way she understands? In her world:
- Hiking and the words left, right, map, and “which way” go together.
- When someone asks a question, you are supposed to answer
More detail:
1. Words Go Together
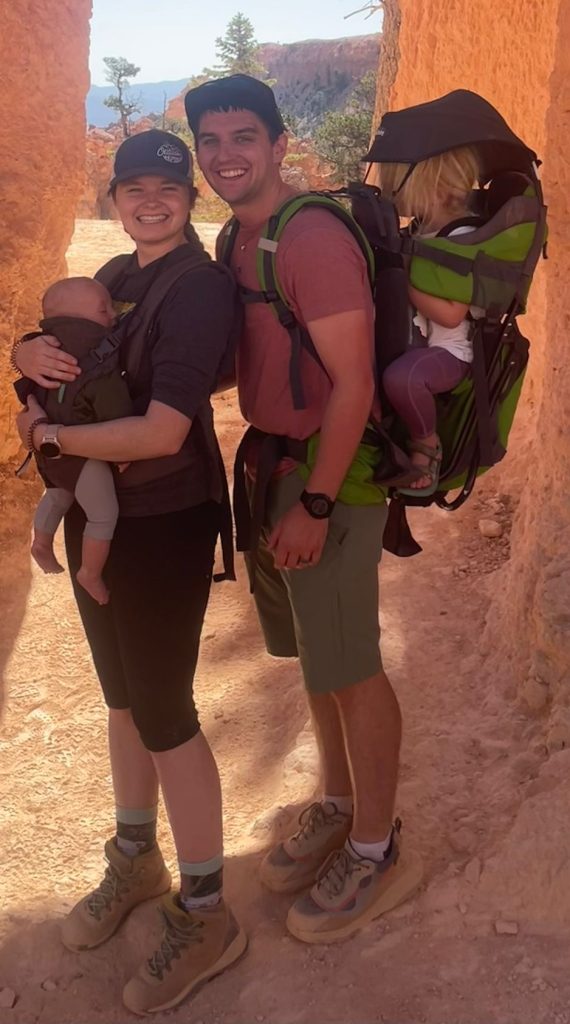
Nora hears grown-ups talk a lot, about a lot of different things. She doesn’t know that she isn’t fully understanding some of those conversations, but she picks up on the word patterns. She has been hiking many times–she has personal experience with it. But her experience is limited: it is always with adults, and she usually is riding in a backpack. Her lived experience with hiking does not include the act of choosing which way to go.
BUT. The grown-ups around her use certain words when they’re on hikes. They say things like, “Which way do we go”, “Let’s look at the map,” and “Oh, it looks like we should take the left fork and then the right fork.” She doesn’t have lived experience with these words, with the act of not knowing where to go and looking it up on a map. She just puts the words together, combines them with other common words, and says something that, on the surface, makes pretty good sense. If an adult were to say it, I would probably believe them.
2. You’re Supposed to Answer
Nora also knows that when someone asks you a question, you’re supposed to answer. “I don’t know” isn’t in her vocabulary. She doesn’t have experience with everything people talk about, but they still talk about it–so she does the same thing. Even though words don’t always have a foundation in her experience, she still strings them together and says them, she still answers questions posed to her.
Large Language Models Also Put Words Together and Always Answer
Like Nora, LLMs put words together, even though they don’t have any real “experience” or “understanding” of what the words mean. They are trained on a type of probability model, putting together the words that went with other words in their training data (data that is a collection of biased human discourse). When trained on huge datasets, they amazingly come up with coherent statements and often get things right.
One difference here is that Nora does have a little bit of experience in the context–she has hiked before. Her first words connected to something concrete–mama, dada. LLMs have no experiences and so no chance of connecting words with the physical or social world. All they do is parrot what has been represented on the internet. Also, Nora will learn and grow; LLMs remain stuck in the no-experience-realm.
But, like Nora, LLMs will answer you, no matter what. Humans, once they reach certain development stages, realize that words always mean something, but LLMs will never get to that point. They don’t “know” that language is supposed to match lived experiences, so do not “know” that they should not just make stuff up. They don’t say “I don’t know” (well, maybe in some cases, they may be trained to do so, but they cannot fully prevent making stuff up–because it isn’t making stuff up, it’s just parroting).
Metaphor 4: ChatGPT and Human Conversations
If Nora’s like ChatGPT–does that mean I’m like its user? Perhaps.
I have some experience with the world that tells me that 3 1/2 year-olds don’t usually understand maps. I also knew that we were probably not going to run into 4 two-way forks on our hike (we were near the end). I knew I shouldn’t follow her directions.
BUT. I did–sort of–make one assumption of “truth”. It seemed like she knew what left and right were. In other words, I dismissed the obvious “hallucinations”–that she read the map–but I did question something that was more plausible.
I have found myself making similar mistakes with LLMs. I know not to accept facts without additional research. But, the other day, when asking for feedback on an activity, I assumed the feedback was based on the activity. But it wasn’t necessarily so–in fact, when investigating further, I found much of it was pretty generic.
What Does this Mean For Using AI?
Our experiences with LLMs are designed to feel like human conversations. They have friendly voices. They are polite and encouraging. They try to please us and keep us around. The result is that it can be really hard to not think and react as if we are talking to a human.
Most reading this post know that GenAI makes stuff up. You know not to trust facts, not to trust academic references, etc. But it is still easy to slip into small assumptions–for example, if it said that it looked something up, does that mean it did? If it says it knows how to calculate an answer, gives the formula, and then gives an answer, does that mean each of those pieces are correct? If it says it is grading student work using a specific rubric, does that mean it is even taking into consideration the work before spitting out a grade? Maybe, maybe not. The more I reflect on my thinking and interactions with LLMs, the more I realize how many small assumptions I make.
This does not mean that LLMs are not useful. They are incredibly useful. But the way we approach them, the way we think when using them, must be very different than anything else we interact with. It requires getting words to think with, not searching for facts. It is an epistemological shift.
A few months ago I wrote a post where I compared this to a legal principle. Hearsay–saying what someone else said–is usually prohibited in trials, but there are exceptions, one of which is when it is not given “for the truth of the matter asserted.” For example, say I’m accused of unaliving my neighbor after they said my cat is ugly. Whether or not my cat is ugly (and she is certainly not ugly) doesn’t matter, if my neighbor said this to me, it could be repeated in court to show that I had murderous motives. Similarly, LLMs can help us without representing the “truth” because they give us some material to consider, but we must use our experience to judge the veracity of that material and the implications of it.
It might be impossible to fully suspend our habit of assuming words have a basis in reality. But, as we are helping others learn to use GenAI, it is critical that we constantly emphasize skepticism, constantly monitor our own thinking, and constantly consider what is different between interacting with it and interacting with a human.
Basic principles I emphasize when using AI–that we should use human agency to direct use, be skeptical, practice metacognition, engage in human dialogue, play and experiment--may help with developing these patterns of thinking. Ultimately it takes a lot of meta-cognitive work to shift our thinking. But if we want to avoid mindlessly perpetuating past discourses–inequitable beliefs, attitudes and social patterns–we must put forth our best effort.