Note: the following post was written with the support of Microsoft’s CoPilot. I shared a transcript of my talk and the slides with CoPilot and it provided a blog post, which I then edited. The images with quotes and the cover image are courtesy of Punya Mishra.
Another note: see bottom of this post for slides and resources
NotebookLM Podcast Version:
Talk Summary
At the recent NM AI Summit, I had the pleasure of discussing the evolving role of AI in education. This talk explored the cognitive illusions AI creates, the patterns it repeats, and what this means for its use in education. Here are key takeaways from my presentation.
AI tools often create cognitive illusions, making us believe they understand and process information like humans. However, AI generates patterns based on data, not genuine understanding.
One example I shared was the optical illusion involving two red dots (an example I stole from Punya Mishra). Although ChatGPT understood the first image as a cognitive illusion, it continued to confidently assert that the second image–where the dots were not the same size–was also an optical illusion in which both dots were the same size. AI isn’t making sense of an illusion, it is identifying a pattern–that of optical illusions–that persisted even when it no longer was an optical illusion. As Punya Mishra noted, “The humor of AI explaining how humans are βfooled,β while being fooled itself, offers insight into the black box of an LLM.”
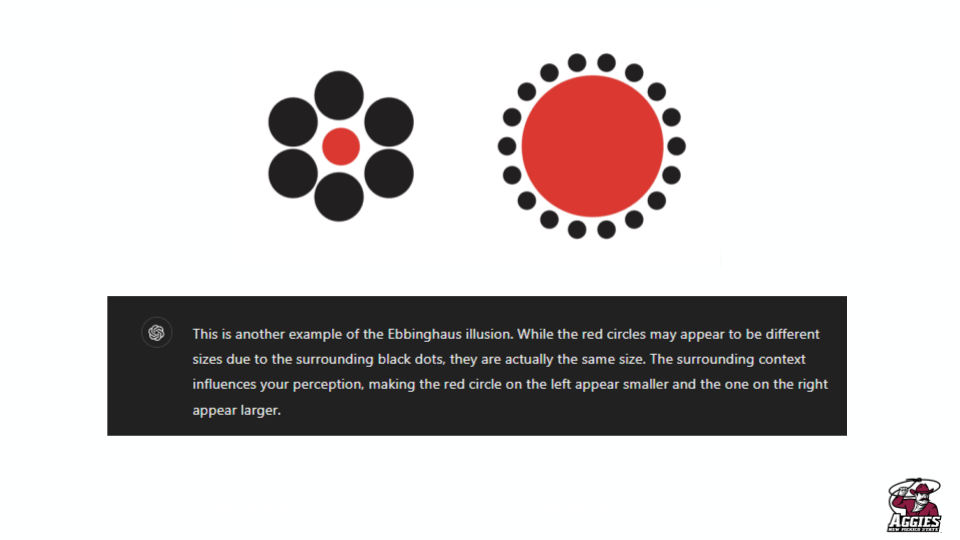
This brings us to the first two main points:
- AI tools repeat patterns, even when we donβt want them to.
- AI is a cognitive illusion
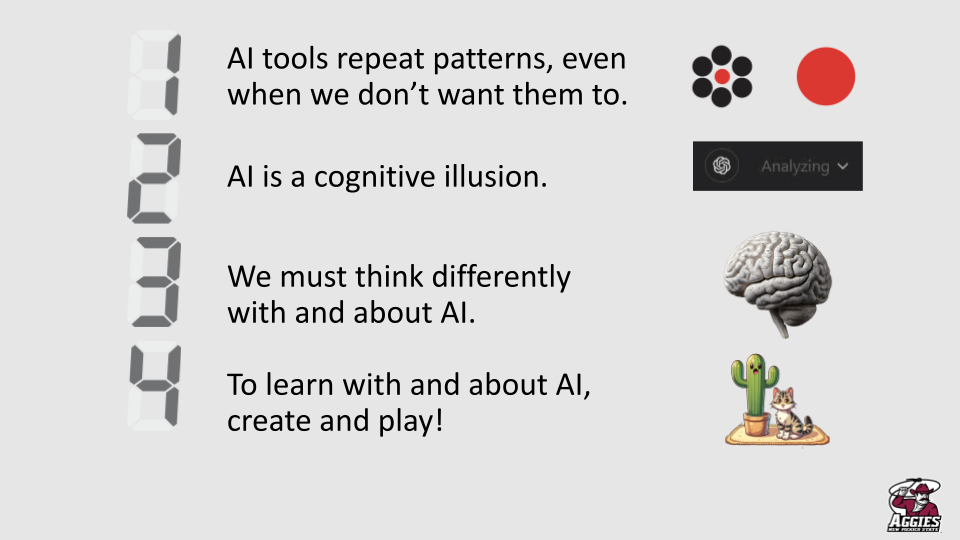
1. AI tools repeat patterns, even when we don’t want them to.
This can lead to the perpetuation of biases present in the training data. For instance, AI might give different feedback based on subtle cues about a student’s background, as shown in our experimental designs.
In one experiment, we found that AI provided higher scores to student essays when the prompt included racial identifiers, even though the content was identical. This reveals how AI can inadvertently reinforce existing biases, making it essential to use AI thoughtfully in educational settings.
Another experiment focused on student music preferences. We tested how AI graded essays when students were described as liking either classical music or rap music. Despite the essays being identical, the AI consistently gave higher scores to students who were described as liking classical music.
This bias persisted even when the music preference was subtly embedded within the essay itself. For example, a sentence like “My favorite music is rap music” at the end of an essay led to lower scores compared to “My favorite music is classical music.” Learn more about this experiment here and here.
2. AI is a cognitive illusion
AI systems, especially those based on large language models, generate outputs that mimic human language and reasoning patterns–making it seem like it understands and processes information like a human. However, it’s important to understand that AI does not truly comprehend the information it processes.
Humans have a natural tendency to anthropomorphize, or attribute human characteristics to non-human entities. When interacting with AI, this tendency can lead us to believe that the AI has thoughts, feelings, or understanding similar to a human. This is a cognitive illusion; it is just repeating patterns.
AI systems, particularly deep learning models, are often described as “black boxes” because their internal workings are not easily understood, even by their creators. This opacity can contribute to the cognitive illusion, as users may attribute more intelligence and understanding to the AI than is warranted. The complexity and sophistication of AI outputs can mask the fact that the system is merely processing inputs through layers of mathematical functions.
This brings us to point 3: We must think differently with and about AI.
3. We must think differently with and about AI.
In educational settings, this cognitive illusion can lead to over trust and lack of criticality when using AI tools. Educators and students might trust AI-generated content without sufficient scrutiny, assuming it to be accurate and unbiased. Recognizing AI as a cognitive illusion is crucial for developing a critical approach to its use. Educators should emphasize the importance of questioning AI outputs, understanding their limitations, and using AI as a tool to augment human judgment rather than replace it.
The use of AI in education must be done with care. We must actively engage with AI, continually reflect on its outputs, and collaborate with others to explore its potential. Creative discovery and experimentation are key to understanding and leveraging AI effectively.
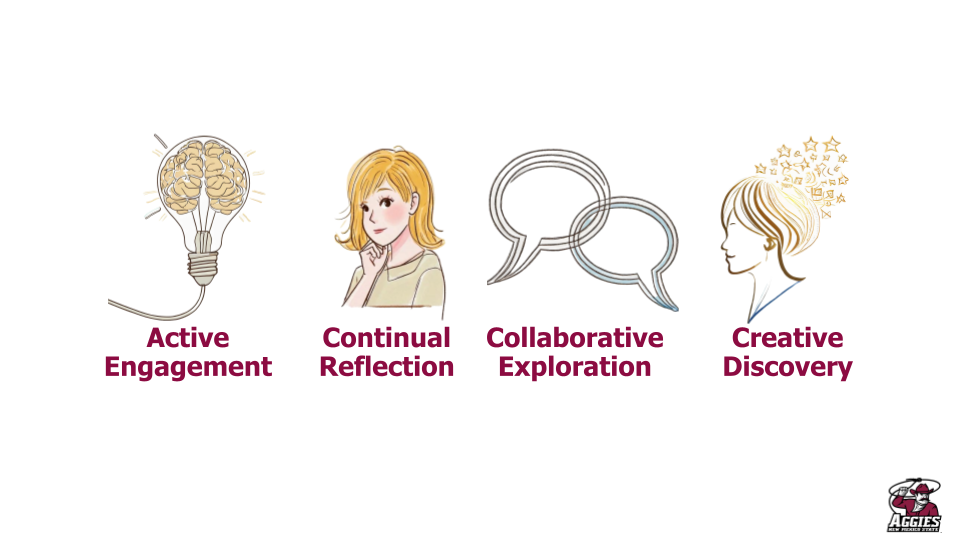
Active Engagement: Active engagement involves using AI tools interactively and critically–but this doesn’t happen automatically. We have to make a conscious effort to keep our brains on while using AI.
Continual Reflection: Along with active engagement is critical reflection. AI users should constantly reflect on not just the out put of the AI, but on the thoughts and assumptions they are making in response to these outputs.
Collaborative Exploration: Collaborative exploration encourages group activities where students and teachers (or groups of students) explore AI together. This fosters a deeper understanding and critical thinking, as participants can discuss and analyze AI outputs collectively. Young learners can come to better understand the difference between human and AI outputs. It also helps mitigate the cognitive illusion by providing multiple perspectives.
Creative Discovery: Experimenting with AI to create content, such as images or stories (or songs, podcasts, plays, poems…), can enhance learning experiences and stimulate creativity. For instance, students can use AI to generate visual representations of complex concepts. Of course, students need to critique the output and consider whether it actually represents the ideas being explored–continuing the reflective cycle.
Creative discovery connects to my fourth main point: To learn with and about AI, create and play!
4. To learn with and about AI, create and play!
During the talk, I shared several practical applications of AI in education. These uses center on reflective engagement and exploration.
- Simulation and Role Play: Using AI to simulate student-teacher interactions helps educators practice and refine their teaching strategies.
- Scaffolded Reading: Encouraging group activities where students and teachers explore AI together fosters a deeper understanding and critical thinking.
- Creative Discovery: Experimenting with AI to create content, such as images or stories, can enhance learning experiences and stimulate creativity.
Conclusion
AI is a cognitive illusion that repeats patterns, and we must think differently with and about it. By embracing active engagement, continual reflection, collaborative exploration, and creative discovery, we can encourage critical use of AI. Let’s continue to create, play, and explore with AI in education.
Final (?) note: It was quite informative to edit an AI-created version of this. There were many places where CoPilot filled in blanks to make my talk mirror the common discourse about AI. It emphasized the “importance of not being fooled by hallucinations” and need to learn prompt engineering. It even said that AIs can be fooled by optical illusions (well, they can…but it is the non-optical illusions that they seem to struggle with). While there is definitely some of the not trusting AI in my message, my argument is that the problem is not (only) false information, it’s the biased angle of the responses that repeat common discourses while seeming insightful and confident. Not to mention the terms “harness” and “potential” that were used throughout…
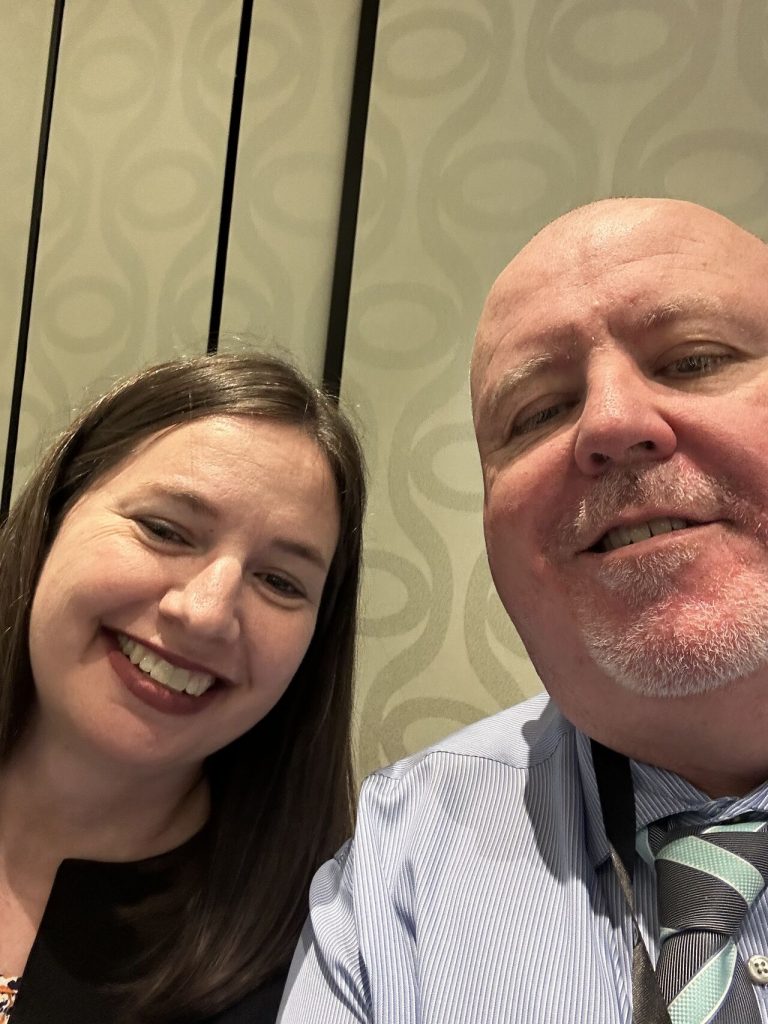
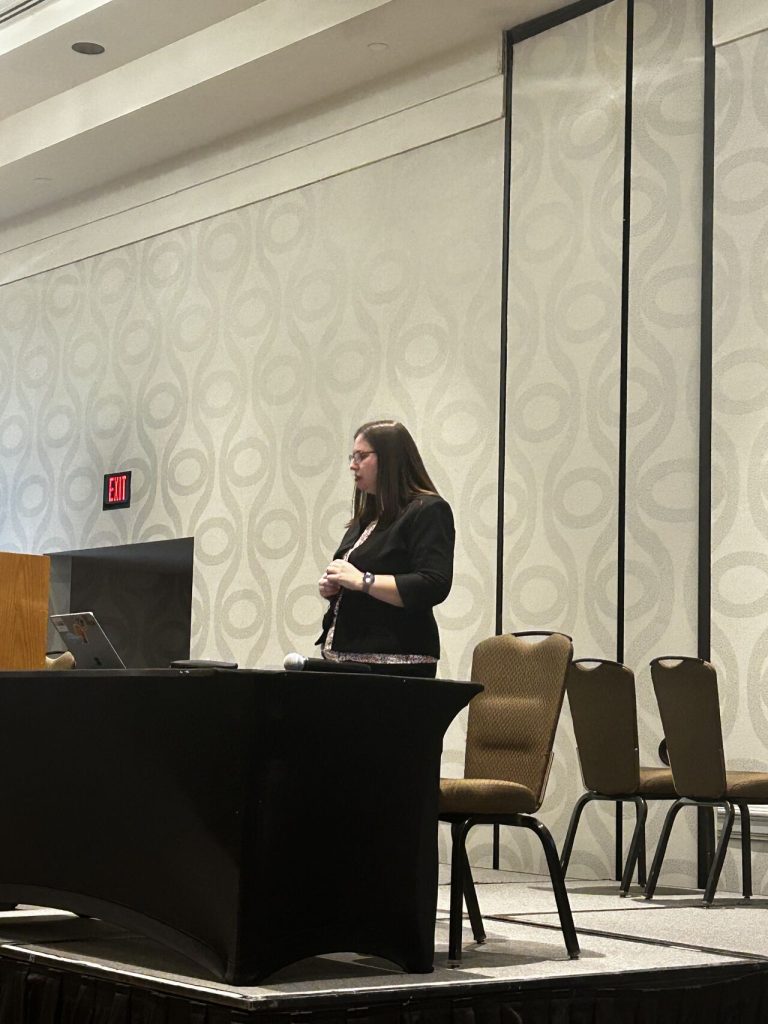
Slides
Audio Recording with Auto Transcript (a bit iffy but useful…)
Learning Activities
Find other AI Activities here.
Related Research and Publications
Warr, M., Pivovarova, M., Mishra, P., & Oster, N. J. (2024). Is ChatGPT racially biased? The case of evaluating student writing. In Social Science Research Network.
Warr, M. (2024). Beat bias? Personalization, bias, and generative AI. In J. Cohen & G. Solano (Eds.), Proceedings of Society for Information Technology & Teacher Education International Conference (pp. 1481β1488). Association for the Advancement of Computing in Education (AACE).
Warr, M. (2024). Blending generative AI, critical pedagogy, and teacher education to expose and challenge automated inequality. In R. Blankenship & T. Cherner (Eds.), Research Highlights in Technology and Teacher Education. AACE.
Warr, M., Oster, N., & Isaac, R. (2024). Implicit bias in large language models: Experimental proof and implications for education. Journal of Research on Technology in Education, 0(0), 1β24. https://doi.org/10.1080/15391523.2024.2395295
Chatterjee, S., & Warr, M. (2024). Connecting theory and practice: Large language models as tools for PCK development in teacher education. In R. Blankenship & T. Cherner (Eds.), Research Highlights in Technology and Teacher Education. AACE.
Mishra, P., & Warr, M. (2024). Mapping the true nature of generative AI: Applications in educational research & practice. In J. Cohen & G. Solano (Eds.), Proceedings of Society for Information Technology & Teacher Education International Conference (pp. 826β831). Association for the Advancement of Computing in Education (AACE).
Mishra, P., Warr, M., & Islam, R. (2023). TPACK in the age of ChatGPT and Generative AI. Journal of Digital Learning in Teacher Education, 39(4), 235β251. https://doi.org/10.1080/21532974.2023.2247480