Note: This post was written with extensive interaction with ChatGPT. You’d think I would know how to fully take advantage of writing with AI by now. But using ChatGPT’s canvas has made a major difference in how I use the tool to really refine my thinking as I write, truly being able to focus almost exclusively on ideas.
I’ve been thinking a lot lately about personalized learning and bias in education. This week I read a bit on the history of visual literacy, giving me a fresh perspective.
I’ve questioned whether AI-supported “personalized learning” is just going to reinforce biased patterns and stereotypes. Some of my research has empirically demonstrated that personalizing with AI can result in inequitable interactions (for example, see here, here, and here and peer-reviewed research here and here). Visual literacy history made me question whether personalized learning is also another way to shape learners to fit dominant norms.
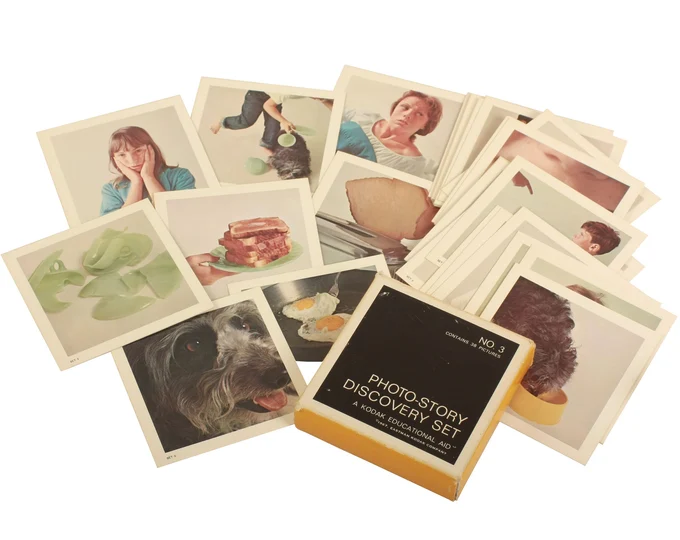
Kodak’s “Photo-Story Discovery Sets” were designed to help children improve their verbal literacy by sequencing pictures. This might sound like a helpful intervention, but it’s important to ask: why was this approach used, and for whom? The program’s target audience was “disadvantaged” children, with an underlying assumption that these children lacked the verbal skills of their peers but could develop these skills through images. In this way, the program’s goal wasn’t just to support children but to assimilate them into a dominant, word-centered form of literacy. It’s a pattern we’ve seen before--in the racist origins of “learning styles” and other deficit-based approaches to education.
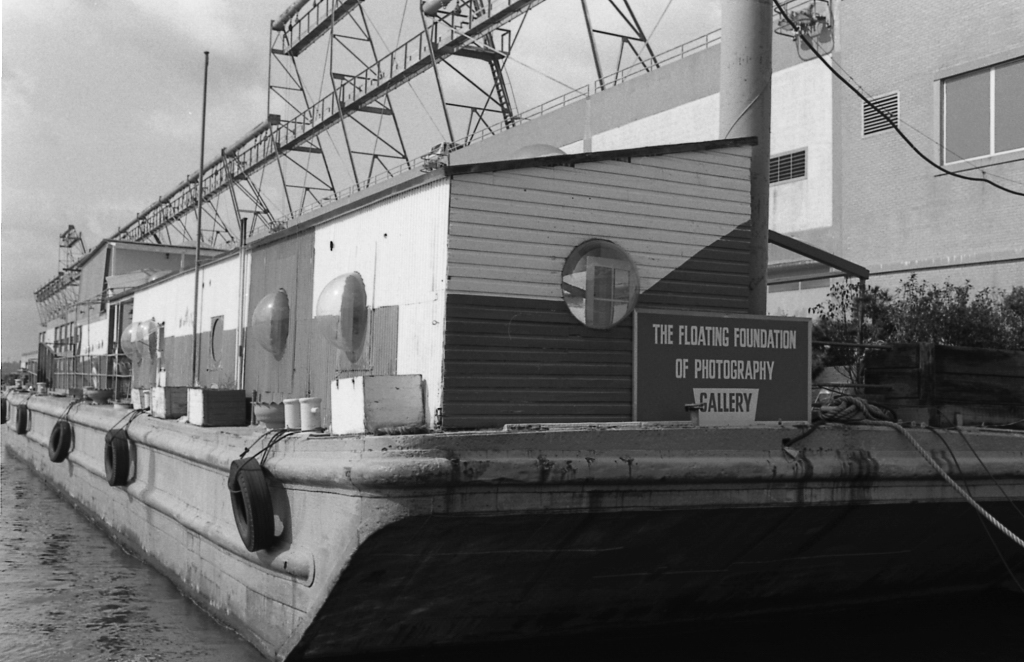
Other visual literacy initiatives took a different approach. Programs like Benedict Fernandez’s Photo-Film Workshop focused on visual communication as a tool for self-representation. Here, the goal wasn’t to “fix” children but to empower them to tell their own stories. Students used photography to make meaning from their own lived experiences. Unlike Kodak’s program, these initiatives didn’t require students to conform to dominant cultural narratives. Instead, they centered the students’ unique perspectives.
So, Will AI-Supported Personalized Learning Reinforce Inequitable Power Structures?
This distinction between assimilation and representation has clear parallels in AI-driven personalized learning. AI-driven personalized learning platforms analyze student performance, identify “gaps,” and guide students toward “competency.” But whose version of “competency” is being used? More often than not, it’s the definition created by those in power. Instead of helping learners use their cultural resources to make meaning of their experiences, these systems may be nudging students to conform to a singular vision of knowing and meaning making.
The cycle could play out like this. First, students—and AI—learn from a world that’s already shaped by dominant cultural norms. Our textbooks, media, and even classroom discussions reflect the perspectives of those with cultural power. Students internalize these norms as they navigate the world. Generative AI models are trained on datasets pulled from the internet—a space that amplifies dominant perspectives. The AI absorbs these norms and feeds them back to students. The learning process now runs on two tracks: one loop from society to learners and AI, and another from AI repeating these ideas back to learners. It’s a closed cycle that leaves little room for alternative perspectives or culturally diverse ways of knowing. More specifically:
- Dominant Ideas Shape AI: AI systems are trained on datasets built from dominant societal norms, narratives, and biases.
- AI Shapes Learners: When students use AI, they are exposed to and internalize its outputs, which reflect these dominant norms. This impacts their thinking, influencing how they process and engage with the world.
- Learners Reinforce AI: As learners internalize these narratives and interact with AI, their behavior and responses further reinforce the dominant ideas embedded in the AI.
- Diminished Engagement with Diverse Viewpoints: Over time, this cycle amplifies dominant perspectives while diminishing learners’ engagement with diverse viewpoints, sidelining reality, personal experiences, and even human interactions.
This pattern risks narrowing learners’ understanding of the world, limiting their ability to engage with diverse viewpoints, and constraining their agency to think critically and creatively about their own experiences.
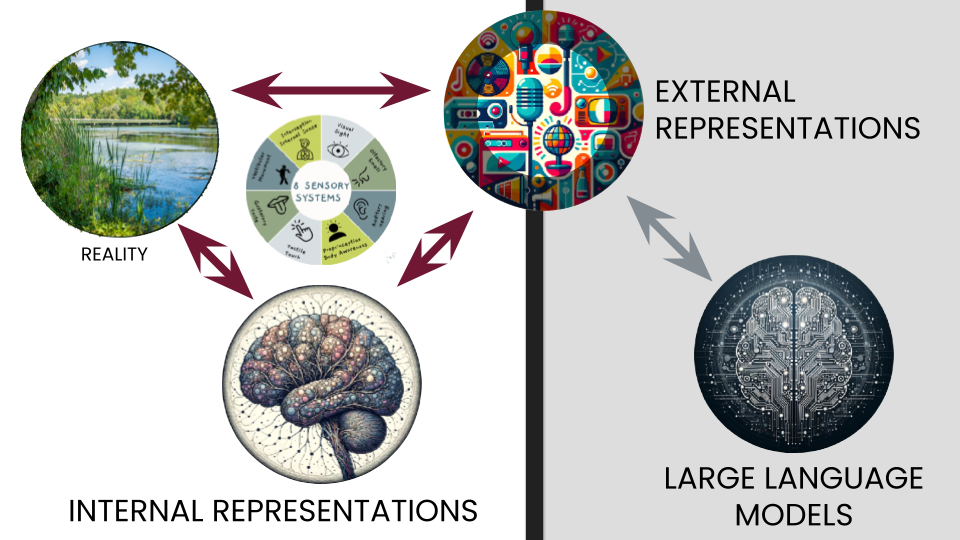
Rather than relying on AI to drive personalized learning, we should encourage learners to be reflective in their interactions with AI and use it as a tool for exploration, critique, and creation. This approach aligns more closely with the second type of visual literacy, where learners actively shape and express their own perspectives.
Instead of having AI systems “personalize” learning for students, we can support students in using AI to tell their own stories, make sense of their lived experiences, and produce new ideas. This requires a shift in focus from passive consumption to active creation, ensuring that learners remain at the center of the process and maintain their cognitive, emotional, and moral agency.